Organizations are recognizing the need to manage their data as products. Traditional data management approaches often separate the management of data assets and data solutions[foot]A data asset is a cohesive set of data that is made readily usable. A data solution is a value-creating initiative that draws on one or more data assets.[/foot] from their respective value realization processes, resulting in illiquid data assets, costly data solutions, and unclear data investment returns. Today, by adopting a product mindset for data, organizations hold people accountable for the payoff of data investments over time. They treat both data assets and data solutions as valuable offerings with a lifecycle—including market development, (internal and external) customer appeal, pricing, deployment, (re)use, continuous improvement, profitability, and retirement. As one MIT CISR Data Research Advisory Board[foot]The MIT CISR Data Research Advisory Board (the "Data Board") is a community of data and analytics leaders from MIT CISR member organizations who participate in and inform MIT CISR research.[/foot] leader put it:
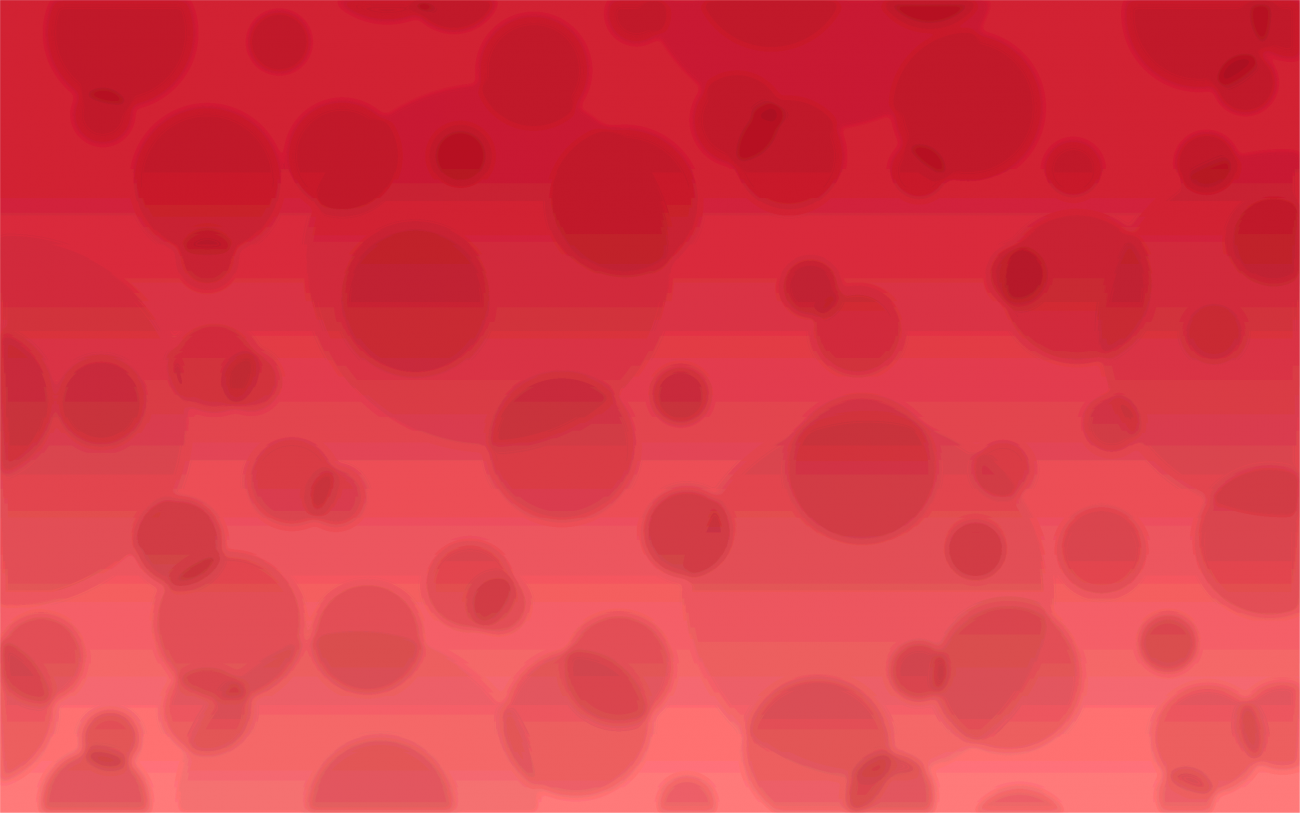
Shifting to a Product Mindset for Data
Abstract
Today, by adopting a product mindset for data, organizations hold people accountable for the payoff of data investments over time. Data assets and data solutions represent two distinct types of “data products.” Organizations can use three sets of product management practices to more effectively generate returns from their data assets and data solutions: practices for satisfying data consumers, practices for setting prices, and practices for sustaining profits. This briefing illustrates these practices using examples from information businesses.
Think about someone responsible for a product that has a profit and loss. They want their product to be used, relevant, and competitive. They think about capabilities that ensure future sustainability. They think about product end of life. Today we bring those concepts into the way that we manage data.
This perspective is prevalent in organizations that rely solely on data for their financial viability, such as information businesses Experian and LexisNexis.[foot]For a description of one information business, see B. H. Wixom, C. M. Beath, I. A. Someh, and J. Duane, “Healthcare IQ: Sensing and Responding to Change,” MIT CISR Working Paper No. 458, February 2023, https://cisr.mit.edu/publication/MIT_CISRwp458_HealthcareIQDataAssets_WixomBeathDuaneSomeh.[/foot] These organizations have succeeded by being great at building competitively distinctive data assets and using them to sell profitable solutions. Our research shows there are three practice areas that contribute to information businesses’ data monetization effectiveness: practices for (1) satisfying data consumers, (2) setting prices, and (3) sustaining profits.[foot]MIT CISR 2024 Data Monetization Survey (N=349). Firms were first aggregated based on strategy archetype responses. Firms with an information business strategy reported significantly higher scores (on a 1–100 scale) than firms with other strategies on satisfying data consumers (61), pricing (65), and sustaining profits (71), all p<.001.[/foot] These practice areas are key elements of product management, and embracing them can help any organization more effectively generate returns from its data assets and data solutions. In this briefing, we describe the practices and demonstrate how organizations of any kind can adapt them to manage their data assets and solutions more effectively, ultimately helping the organization realize its strategic business outcomes.[foot]For a description of strategic business outcomes, which MIT CISR has termed data monetization impact, see N. van der Meulen, B. H. Wixom, and C. M. Beath, “Maximizing Returns from Data Monetization Strategies,” MIT CISR Research Briefing, Vol. XXV, No. 2, February 2025, https://cisr.mit.edu/publication/2025_0201_DataMonetizationImpact_vanderMeulenWixomBeath.[/foot]
Two Kinds of Data Products
Data assets and data solutions represent two distinct types of data products. Both benefit from core product management principles, but their application of the principles differs. Understanding the difference is crucial for maximizing data monetization impact.
A data asset is an organizational resource, a cohesive set of data that is made readily usable for data consumers. Data asset owners are data source and data domain experts that have a vested interest in an asset’s care and feeding. They manage service-level goals associated with data integrity and usability, such as data quality, standardization, and latency, and they measure use and reuse. Their target customer is the data asset consumer, such as a data power user or the owner of a data solution.
A data solution is an application, dashboard, website, system, or even a set of APIs that delivers specific functionality to users by consuming one or more data assets. Data solution owners typically begin their role as the business leads for specific value-creating initiatives. They steer development to meet specified user needs and ensure adoption, and they set metrics regarding solution-specific value creation (i.e., benefits) and value realization (i.e., cost savings and revenues) to drive value. Their target customer is the end user or beneficiary of the data solution.
Notably, data assets and data solutions are highly interdependent. Data assets get used when they contain information fit for data solution purposes. A poorly managed or illiquid data asset slows down or weakens solutions that depend on it. But the availability of liquid data assets leads to experimentation and innovation. Consider the case of the multinational beverage, retail, and logistics company FEMSA. In 2023, the company developed a dead net profit (DNP)[foot]DNP is a financial metric that calculates SKU-level revenues and costs, accounting for every business activity, to arrive at a true measure of profit.[/foot] data asset that made SKU-level profitability transparent at all levels of the company’s OXXO convenience store chain.[foot]R. V. Bradley, B.H. Wixom, and C. M. Beath, “Internal Data Sharing: Four Examples,” MIT CISR Working Paper No. 459, May 2023, https://cisr.mit.edu/publication/MIT_CISRwp459_DataSharingExamples_BradleyWixomBeath.[/foot] Since the asset’s deployment, OXXO has reused it to create data solutions for store management, merchandising, assortment, and operations.
Enabling Product Management Practices
Product management practices help owners of data assets and data solutions sell, improve, and profit from their respective products. The following sections describe three key practice areas, illustrated using examples from information businesses.
Practices for Satisfying Data Consumers
Satisfying consumers of data assets and data solutions (whether internal or external, and prospective or current) requires a deep understanding of their pain points and evolving needs. It relies on an advanced customer understanding capability: the ability to gather accurate and actionable knowledge about customer needs and behaviors, then to cocreate solutions and test hypotheses about preferences.[foot]Barbara H. Wixom, Cynthia M. Beath, and Leslie Owens, Data is Everybody’s Business: The Fundamentals of Data Monetization (The MIT Press, 2023), https://cisr.mit.edu/publication/data-everybodys-business-book.[/foot]
Information businesses develop advanced customer understanding by testing new ideas with customers—for instance, using A/B testing or controlled field experiments—and by using behavioral analytics to analyze customer usage patterns, uncover core and latent needs, and remediate issues. For example, the organizational unit at global media metrics company comScore that was dedicated to supporting the uptake of the company’s data solutions[foot]B. H. Wixom, J. W. Ross, and C. M. Beath, “comScore, Inc.: Making Analytics Count” MIT CISR Working Paper No. 392, November 2013, https://cisr.mit.edu/publication/MIT_CISRwp392_comScore_WixomRossBeath.[/foot] tracked actual usage of solutions at comScore’s client companies and actively encouraged clients to use the solutions they had purchased. Other information businesses establish business development units, to identify new high-potential markets and determine when and how their data assets and data solutions need to be enhanced or changed to meet prospective customer needs.
Practices for Setting Prices
Setting effective prices for data assets and solutions requires a deep understanding of their profit formula, which includes costs, desired margin, and the consumer’s willingness to pay. Information business leaders know that data solutions must collectively fund the cost of developing and sustaining the reusable data assets on which those solutions rely. For example, a data asset in one information business from our research was used for two solutions: one had high transaction volume, and that solution was priced at pennies per transaction. The other was a critical solution for a single client, and it was priced at hundreds of thousands of dollars. Each solution had a different profit formula, but together they earned enough to fund continuous improvement of the data asset.
For cost understanding of data assets, information businesses track and allocate the costs of data acquisition, storage, processing, and maintenance. Often, they use chargeback accounting to charge solution owners for data asset usage, promoting judicious usage and cost awareness. The remaining costs of a data solution are easier to understand. Information businesses set desired margins for solutions by factoring in the company’s cost of capital and its profit expectations. For assessing the customer’s willingness to pay for a solution, information businesses establish beta programs for solutions (or give away free prototypes) to generate early feedback on value and pricing; to predict willingness to pay for an asset, they offer consulting services to learn how data assets might solve customer problems and how valuable those solutions might be.
When possible, information businesses use instrumentation to expose important parts of their profit formula. For example, the travel expense management company TRIPBAM instrumented its solutions to surface clients’ cost savings, ensuring each client saved at least four times the cost of TRIPBAM’s solution. This transparency allowed TRIPBAM to monitor value delivery and adjust pricing.[foot]G. Piccoli, F. Pigni, J. Rodriguez, and B. H. Wixom, “TRIPBAM: Creating Digital Value at the Time of the COVID-19 Pandemic,” MIT CISR Working Paper No. 444, July 2020, https://cisr.mit.edu/publication/MIT_CISRwp444_TRIPBAM_PiccoliPigniRodriguezWixom.[/foot]
Practices for Sustaining Profits
Sustaining profits from data assets and solutions requires organizational agility: the ability to sense and seize opportunities that come from changes in the organization’s environment. This involves continuously adapting data solutions and their underlying data assets to changing market needs, customer demands, and competitive threats, while keeping costs in check.
To stay agile, information businesses staff people in reciprocal roles, customer-facing roles with the responsibility to continuously make sense of customer needs and initiate changes in both data assets and data solutions. At Healthcare IQ’s customer care center (staffed to assist users of self-service solutions), care center staff not only addressed the immediate needs of solution end users but also identified opportunities for improvements in solutions and assets. For example, when the US government introduced new value-based care legislation that pressured hospitals to cut costs, customers of Healthcare IQ’s cost management solutions started asking new questions about cost savings. Care center employee input prompted Healthcare IQ leaders to invest in adding clinical data to the company’s hospital supply chain data asset and to add new solution features.
Information businesses also defensively protect their intellectual property to forestall erosion of value. In the case of Healthcare IQ, the CEO acquired an organization that held the patents to a technology underlying the company’s core product line, proactively fending off potential competitors.[foot]B. H. Wixom and C. A. Miller, “Healthcare IQ: Competing as the ‘Switzerland’ of Health Spend Analytics,” MIT CISR Working Paper No. 400, February 2015, https://cisr.mit.edu/publication/MIT_CISRwp400_HealthcareIQ_WixomMiller.[/foot]
In sum, organizations can use product management practices to better manage their data assets and data solutions. Table 1 describes how each practice differs for the two products.
Table 1: Data Asset and Data Solution Differences per Practice Area
|
Data Asset | Data Solution |
---|---|---|
Satisfying consumers | Data asset teams interact with solution teams to learn how to build and evolve data assets that appeal to new and existing users. They spark the imagination of new users, developers, or champions to drive data asset reuse. | Data solution teams manage the needs of end users by developing advanced customer understanding capabilities for cocreating, experimenting, and performing customer analytics on value creation. |
Setting prices | Data asset owners use indirect pricing methods; some recoup costs with transfer pricing based on use or customer value created. | Data solution owners use direct pricing for external solutions and indirect pricing for internal solutions or periodic calculation of value. |
Sustaining profits | The organizational ability to track and manage the creation of data assets and other data resources Data asset owners instrument and track data asset use. They rely on solution owners to track value creation and realization, and adapt and enhance data assets to sustain use and encourage reuse. | Data solution owners control costs and continuously adapt the solution to changing market needs and competitive threats, in part by requesting complementary changes to underlying data assets. |
Beyond Stewardship: Owning Data’s Value
Organizations that are already familiar with product management for their data solutions are poised to apply a product mindset to the effective management of their data assets. To start, they need to identify broadly relevant and strategically important data that will naturally generate higher initial demand and achieve greater reuse. They may designate owners for those data assets and instrument them to allow the tracking of data asset usage, costs, and value. Data asset owners need to have sufficient visibility into how their assets are used to meet customer needs, as well as into the profitability of resulting solutions, to know when and where to invest in their assets. It is by implementing these practices that you’ll shift from managing data to data product management—and unlock the full potential of your data assets.
© 2025 MIT Center for Information Systems Research, Wixom, Van der Meulen, and Beath. MIT CISR Research Briefings are published monthly to update the center’s member organizations on current research projects.
About the Researchers
MIT CENTER FOR INFORMATION SYSTEMS RESEARCH (CISR)
Founded in 1974 and grounded in MIT's tradition of combining academic knowledge and practical purpose, MIT CISR helps executives meet the challenge of leading increasingly digital and data-driven organizations. We work directly with digital leaders, executives, and boards to develop our insights. Our consortium forms a global community that comprises around seventy organizations.
MIT CISR Associate Members
MIT CISR wishes to thank all of our associate members for their support and contributions.