Artificial intelligence (AI) has been described as everything from just another technology to the beginning of the end of civilization. With so much excitement and hype around AI, we pursued research that would help leaders make sense of the chaos and understand how enterprises create value with AI. We identified four stages of enterprise AI maturity, based on our October 2022 survey of 721 companies. We found that financial performance improved with each stage, and pinpointed capabilities an enterprise needs as it progresses through the stages. The bottom line was that enterprises in the first two stages of AI maturity had financial performance below industry average, while enterprises in stages 3 and 4 had financial performance well above industry average. These findings provided the basis for our MIT CISR Enterprise AI Maturity Model. Given when we conducted the survey, the results primarily describe the relationship between use of analytical (aka traditional) AI and financial performance. To supplement the survey findings, in 2024 we interviewed sixteen executives at nine enterprises about both traditional and generative AI and their early thoughts on agentic and robotic AI.[foot]The data underlying the MIT CISR Enterprise AI Maturity Model comes from the MIT CISR 2022 Future Ready Survey (N=721). We divided respondents into four stages of AI Capability using a measure of Total AI Effectiveness, the equally weighted combination of three measures: effectiveness of AI to (i) improve operations, (ii) improve customer experience, and (iii) support and develop the ecosystem. On a 0–100% scale of Total AI Effectiveness, Stage 1 AI Capability=0–49%, Stage 2=50–74%, Stage 3=75–99%, and Stage 4=100%. We supplemented the model with sixteen interviews we conducted in August to November 2024 with senior executives at nine enterprises.[/foot]
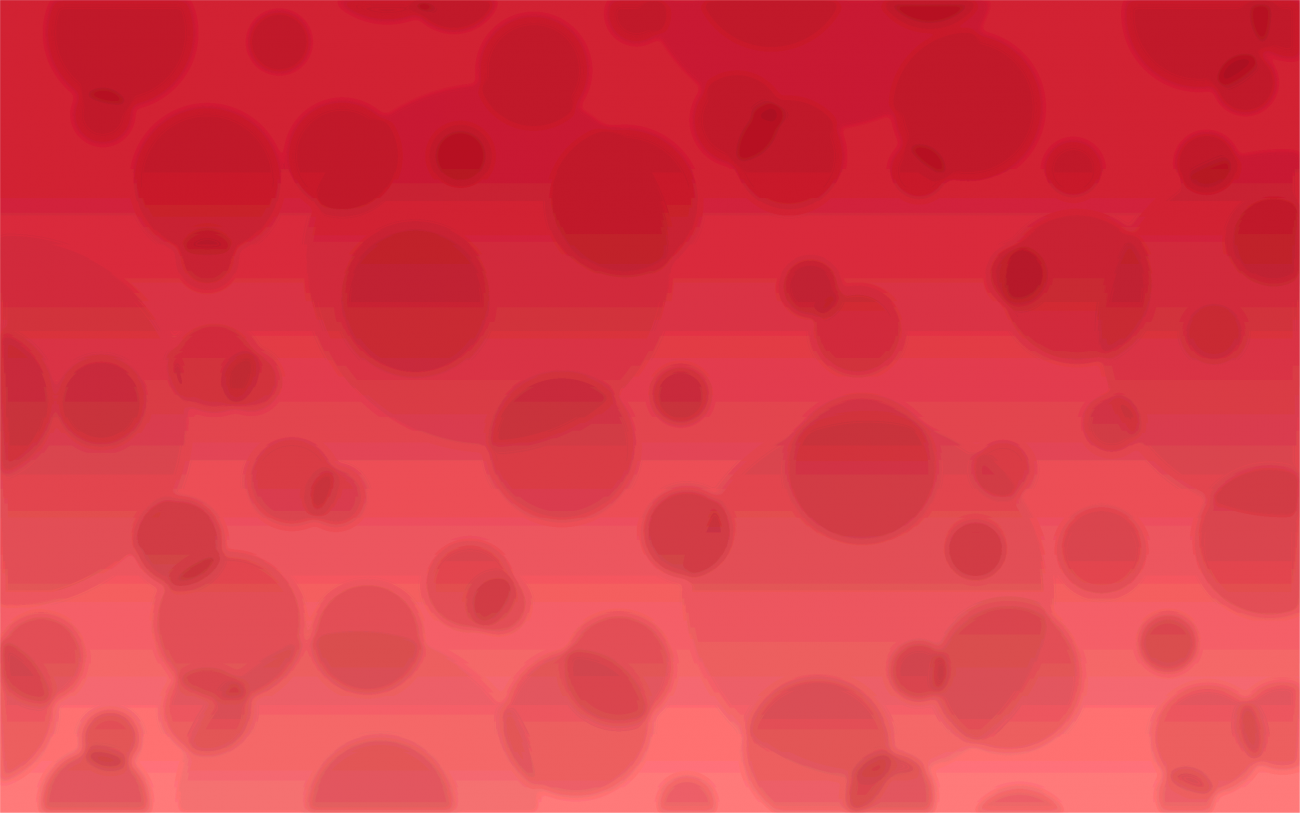
Building Enterprise AI Maturity
Abstract
With so much excitement and hype around AI, we pursued research that would help leaders make sense of the chaos and understand how enterprises create value with AI. This briefing describes the MIT CISR Enterprise AI Maturity model, which depicts four stages of enterprise AI maturity we identified based on a 2022 MIT CISR survey of 721 companies. We found that financial performance improved at each stage, and we pinpointed capabilities an enterprise needs as it progresses through the stages.
Four Stages of Enterprise AI Maturity
Enterprises need to cumulatively build capabilities and learnings from AI as they move toward a future-ready state of AI use. Each of the four stages of the enterprise AI maturity model describes what enterprises focus on in that stage (see figure 1).
Figure 1: The MIT CISR Enterprise AI Maturity Model
AI Stage Attributes | Stage 1: Experiment and Prepare | Stage 2: Build Pilots and Capabilities | Stage 3: Develop AI Ways of Working | Stage 4: Become AI Future Ready |
---|---|---|---|---|
Percentage of Responding Enterprises |
28% | 34% | 31% | 7% |
Characteristics |
|
|
|
|
Focus | Exploration and Education | Business Cases and Pilots | Scaling AI Platforms and Dashboards | Continuous Innovation and New Revenue Streams |
Growth | -12.6 pp | -3.5 pp | +11.3 pp | +17.1 pp |
Profit | -9.6 pp | -2.2 pp | +8.7 pp | +10.4 pp |
Source: MIT CISR 2022 Future Ready Survey (N=721) and interviews in 2024 with sixteen executives at nine enterprises. Respondents were grouped into four stages using a measure of Total AI Effectiveness, the equally weighted combo of three measures: effectiveness of AI to (i) improve operations, (ii) improve customer experience, and (iii) support and develop the ecosystem. On a 0–100% scale of Total AI Effectiveness, Stage 1 AI Capability=0–49%, Stage 2=50–74%, Stage 3=75–99%, Stage 4=100%. Measures of growth and profit are from 2022, calculated as percentage points (pp) above/below industry average.
Stage 1: Experiment and Prepare
The first stage of developing enterprise AI maturity is about preparing and experimenting to use AI effectively. In our survey analysis, 28 percent of enterprises were in this stage. Stage 1 enterprises focus on educating their workforce, formulating AI policies, becoming more evidence-based, and experimenting with AI technologies to get more comfortable with automated decision-making. They discuss where humans need to be in the loop for oversight and what is acceptable and ethical use of AI technology. Funding targets AI literacy for the board and top management team, and skill building on AI technologies integrated into enterprise software for the rest of the enterprise. During stage 1, the enterprise begins to identify both value creation opportunities from AI and the enterprise capabilities and competencies required to realize them.
For example, Kaiser Permanente, a US West Coast not-for-profit healthcare enterprise with 12.5 million members,[foot]“Fast Facts,” Who We Are, Kaiser Permanente, https://about.kaiserpermanente.org/who-we-are/fast-facts.[/foot] defined seven principles for assessing its responsible use of AI: privacy, reliability, high-quality and affordable outcomes, transparency, equity, customer prioritization, and trust. These principles help Kaiser Permanente ensure that AI tools meet its standards.[foot]Daniel Yang, MD, “AI in health care: 7 principles of responsible use,” Kaiser Permanente, August 19, 2024, https://about.kaiserpermanente.org/news/ai-in-health-care-7-principles-of-responsible-use.[/foot]
Stage 2: Build Pilots and Capabilities
The second stage, representing 34 percent of enterprises in our research, is about setting up AI pilots to demonstrate AI’s ability to create value for both the enterprise and its people. In this stage, enterprises define important metrics, begin to simplify and automate business processes, and develop the enterprise capabilities they learned they need during the last stage. Stage 2 enterprises focus on moving from experiments to systematic innovation by piloting use cases, tracking value created in the pilots, and storytelling both internally and externally about learnings from the pilots. Fundamental to stage 2, the enterprise determines how to consolidate organizational data silos and safely and securely serve the data for use with AI; this typically requires a significant investment in, or refinement of, APIs that link the data and the technologies.
In an AI pilot, the disability underwriting team at Guardian Life, a US mutual insurance enterprise, is working with a partner to develop a generative AI tool that summarizes documentation and augments decision-making. Participating underwriters are saving on average five hours per day, building on Guardian’s rich internal data and automated processes. This pilot is helping Guardian to achieve its goal of reimagining end-to-end process transformation.[foot]Used with permission.[/foot]
Stage 3: Develop AI Ways of Working
Moving to stage 3 is a big step in the AI journey, both in terms of potential impact on enterprise growth and profitability and the platform capabilities needed to achieve the scale required. Stage 3, which represented 31 percent of enterprises in our research, is focused on industrializing AI throughout the enterprise. This includes building a scalable enterprise architecture (a platform for AI that allows for scaling and reusing models), making data and outcomes transparent via business dashboards, developing a pervasive test-and-learn culture, and expanding business process automation efforts.
Ally, the largest all-digital bank in the US, is scaling AI via its Ally.ai platform, which the enterprise launched in July 2023. The platform houses Ally AI tools that leverage technologies including natural language processing, machine learning, predictive analytics, and computer vision, and integrates several commercially available large language models with the enterprise’s data in a safe environment. Early value from generative AI use cases includes saving an average three minutes per customer call on more than four million customer calls in 2023 using call summarization, and developing creative campaigns 34 percent faster using a marketing generative AI chat feature.[foot]Used with permission.[/foot]
In stage 3 we see enterprises making significant use of foundation models, and small language models (SLMs) that are trained on an industry, a function, or to perform specific tasks (such as onboarding a customer), to advance their journeys.[foot]Foundation models are a type of AI technology trained on vast amounts data that can be adapted to perform a wide variety of tasks. The models can be fine-tuned for specific domains or tasks by incorporating smaller, specialized, curated datasets into the training set or by using software plug-ins or add-ons. Large language models (LLMs) are general-purpose foundation models. See Ben Lutkevich, “Foundation models explained: Everything you need to know,” TechTarget, April 17, 2024, https://www.techtarget.com/whatis/feature/Foundation-models-explained-Everything-you-need-to-know. Small language models (SLMs) are typically trained on smaller data sets tailored to specific industries, tasks, or domains and contain far fewer parameters. SLMs struggle when it comes to general knowledge and understanding but usually have less bias. See “LLMs vs SLMs,” The Red Hat Blog, October 1, 2024, https://www.redhat.com/en/topics/ai/llm-vs-slm.[/foot] They take these foundation models and SLMs and, on secure enterprise platforms, apply them to their own data to create and capture new value.
Stage 4: Become AI Future Ready
In the fourth stage enterprises are what we call AI future ready, which represented 7 percent of enterprises in our research. AI is embedded in all decision-making in these enterprises. They leverage proprietary AI[foot]We define proprietary AI as the AI infrastructure developed in-house. For instance, an AI platform developed in-house that integrates purchased large language models and enterprise assets such as APIs, data, and business processes is proprietary AI.[/foot] internally, and many sell new business services based on that capability, the AI capability as a service, or both to other enterprises.
Ping An Insurance, one of the largest financial services enterprises in China, debuted an AI banking platform in 2019[foot]“AI Banker: Reshaping Retail Banking for the Digital Age,” Ping An Group, August 26, 2019, https://group.pingan.com/media/perspectives/AI-Banker-Reshaping-Retail-Banking-for-the-Digital-Age.html.[/foot] and is now leveraging it. By the end of 2022, 49 percent of the enterprise’s product sales were made by AI service representatives, and 82 percent of service interactions were handled by AI representatives (i.e., AI software agents[foot]An AI or intelligent agent is a type of AI software that can understand and respond to its environment without human intervention. A smart thermostat is an example of an AI agent, as is a chatbot that can answer questions, or a piece of software that can make decisions. AI agents can adapt based on experience. See Cameron Hashemi-Pour, “What is an intelligent agent?,” TechTarget, https://www.techtarget.com/searchenterpriseai/definition/agent-intelligent-agent, accessed December 2, 2024.[/foot]).[foot]Ping An, 2022 Annual Results, March 2023, https://group.pingan.com/resource/pingan/IR-Docs/2023/pingan-ar22-presentation.pdf.[/foot] Also by the end of that year, AI representatives had saved RMB 600 million in labor costs[foot]“Ping An Bank Chief Information Officer: Technology Supports Sustainability of Finance,” PR Newswire, June 30, 2023, https://www.prnewswire.com/apac/news-releases/ping-an-bank-chief-information-officer-technology-supports-sustainability-of-finance-301867764.html. RMB 600 million equals approximately US$83 million (currency conversion from Google Finance on November 26, 2024).[/foot] for the enterprise, which additionally provided AI banking as a service to thirty other Chinese banks.[foot]“In the field with Ping An,” IMD, November 2020, https://www.imd.org/research-knowledge/digital/articles/in-the-field-with-ping-an/.[/foot] In 2023, Ping An Group reported increased sales, reduced costs, improved efficiency, and reduced risks from using generative AI applications.[foot]Ping An, 2023 Annual Results, March 2024, https://group.pingan.com/resource/pingan/IR-Docs/2024/pingan-ar23-presentation.pdf.[/foot]
In our 2024 interviews as part of this research, an emerging theme was that interviewees expected the most value from AI will be created from combining people and platforms with a combination of four types of AI: analytical, generative, agentic and robotic.
Your AI Maturity Stage Today
We recommend that you bring a team together to talk about which of the four stages your enterprise is in today, and your aspirations and time frames regarding your enterprise’s use of AI. Then, discuss which enterprise capabilities and skill sets need more work.
No matter where you are in the MIT CISR Enterprise AI Maturity Model, be bold. For example, Piyush Gupta, the CEO of DBS Bank, set a KPI of conducting one thousand experiments per year.[foot]Thomas H. Davenport and Randy Bean, “Portrait of an AI Leader: Piyush Gupta of DBS Bank,” MIT Sloan Management Review, August 31, 2021, https://sloanreview.mit.edu/article/portrait-of-an-ai-leader-piyush-gupta-of-dbs-bank/.[/foot] DBS is building on that experimental mindset, coupled with a years-long investment in a data foundation, to become an AI-fueled bank, with AI pervasive across the enterprise. From 2022 to 2024, the bank doubled the economic impact achieved from AI from S$150 million to S$370 million. DBS has delivered over three hundred fifty AI use cases to date, and expects the economic impact of these to exceed S$1B in 2025.[foot]S. K. Sia, P. Weill, and M. Xu, “DBS: From the ‘World’s Best Bank’ to Building the Future-Ready Enterprise,” MIT CISR Working Paper No. 436, March 2019, https://cisr.mit.edu/publication/MIT_CISRwp436_DBS-FutureReadyEnterprise_SiaWeillXu; Aaron Tan, “How DBS is industrialising AI across its business,” Computer Weekly, February 14, 2024, https://www.computerweekly.com/news/366569993/How-DBS-is-industrialising-AI-across-its-business; “Harvard Business School examines DBS’ AI strategy and implementation in its first case study focusing on AI in an Asian bank,” PR Newswire, September 16, 2024, https://www.prnewswire.com/news-releases/harvard-business-school-examines-dbs-ai-strategy-and-implementation-in-its-first-case-study-focusing-on-ai-in-an-asian-bank-302248738.html. S$1 billion equals approximately US$740 million (currency conversion from Google Finance on November 26, 2024).[/foot] What are your tangible goals for AI, and how will you get there?
© 2024 MIT Center for Information Systems Research, Weill, Woerner, and Sebastian. MIT CISR Research Briefings are published monthly to update the center’s member organizations on current research projects.
About the Researchers
MIT CENTER FOR INFORMATION SYSTEMS RESEARCH (CISR)
Founded in 1974 and grounded in MIT's tradition of combining academic knowledge and practical purpose, MIT CISR helps executives meet the challenge of leading increasingly digital and data-driven organizations. We work directly with digital leaders, executives, and boards to develop our insights. Our consortium forms a global community that comprises around seventy organizations.
MIT CISR Associate Members
MIT CISR wishes to thank all of our associate members for their support and contributions.