Since the 1990s, companies have leveraged systems, processes, people, and data to be more efficient and cut costs. At MIT CISR, we describe this as the journey to become “digitized,” and we refer to data activities that help digitized companies save money as “data monetization by improving.” For decades, we have studied digitized companies that have saved millions of dollars by monetizing their data in this way. Examples of these data activities have been varied, such as using production data to predict and remediate operational breaks, and analyzing customer data to better target lucrative market segments. Data monetization by improving is usually a safe pursuit because (1) its focus is internal operations, which is a domain that company leaders can best understand and control, and (2) its participants are employees who are fairly forgiving when data quality or analytics delivery levels fall short. On average, companies today generate 51 percent of their overall data monetization returns by improving.[foot]From the 2018 MIT CISR Data Monetization Survey, N=315. The survey was administered to executives familiar with the company’s enter-prise-level data activities and outcomes. See more study detail and the survey findings in B. H. Wixom, “Data Monetization: Generating Financial Returns from Data and Analytics—Summary of Survey Findings,” MIT Sloan CISR Working Paper No. 437, April 2019.[/foot]
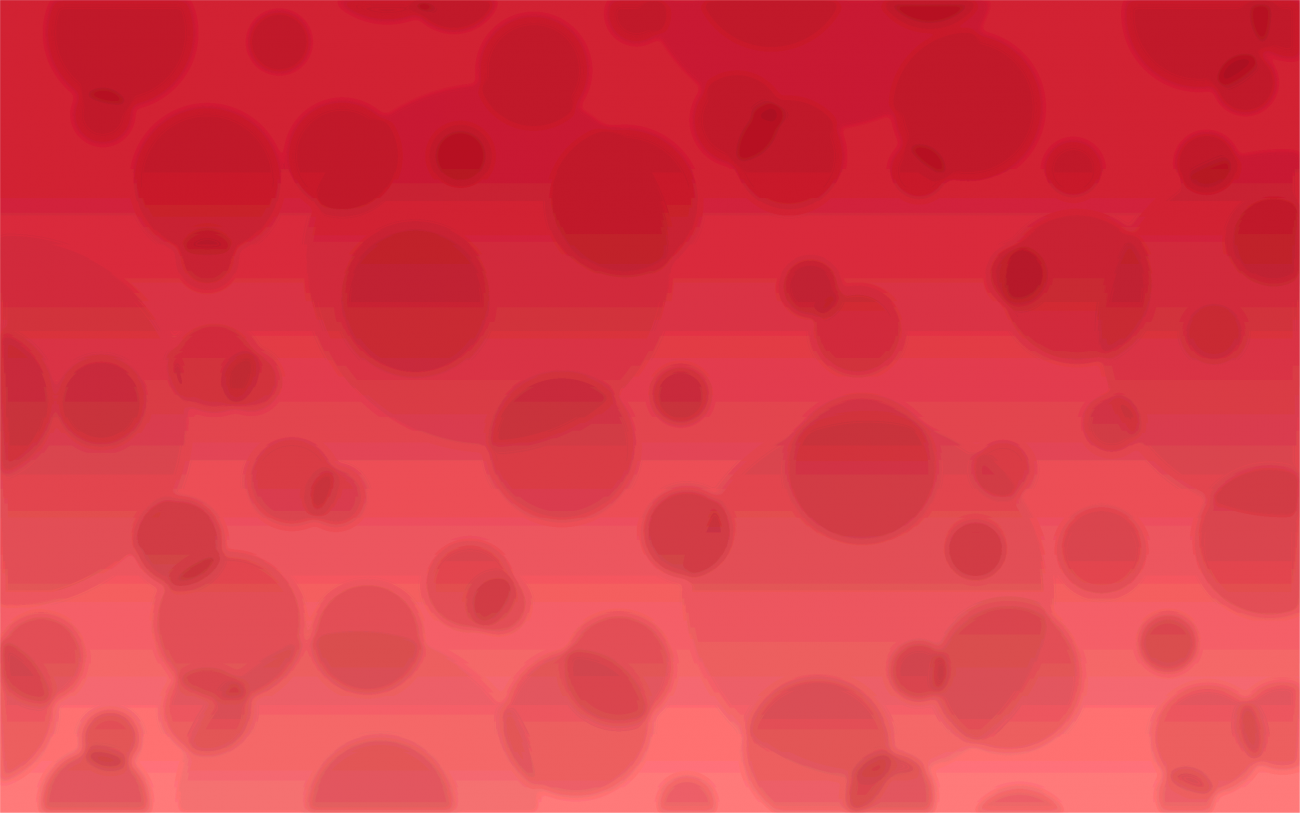
Digital Data Monetization Capabilities
Abstract
In 2018, MIT CISR collected information from 315 executives to learn how companies are generating economic returns from data monetization activities. What emerged from this study is the clear evidence that firms with high levels of five capabilities—data asset curation, data factory platform, data science techniques and talent, customer understanding, and acceptable data use—produce optimized data monetization portfolios. We believe these capabilities allow digital firms to more successfully deploy data and analytics products, features, and experiences that are valued by customers, profitable, competitively superior, innovative, and first to market.
Digital data monetization occurs when companies generate direct or indirect economic returns from market-facing, analytics-based products, features, or experiences.
Companies generate the other 49 percent of their data monetization returns from delivering analytics products, features, and experiences to the marketplace.[foot]We distinguish market-facing data monetization as wrapping and selling. See Barbara H. Wixom and Jeanne W. Ross, “How to Monetize Your Data,” MIT Sloan Management Review, January 9, 2017.[/foot] Established companies entertain the idea of market-facing data monetization as they redesign to become more like Facebook and Spotify. (At MIT CISR, we describe this redesign as the journey to become “digital.”)[foot]J. W. Ross, C. M. Beath, and I. M. Sebastian, “Digitized ≠ Digital,” MIT Sloan CISR Research Briefing, Vol. XVII, No. 10, October 2017.[/foot] Leaders at established companies, however, express both awe and fear as they track the innovation wins and missteps of the digital companies they seek to emulate. Simply put, these leaders want to deliver fruitful market-facing digital offerings[foot]Digital offerings add to the value of a company’s existing product and service portfolio by leveraging the capabilities of digital technologies to solve customer problems. See J. W. Ross, C. M. Beath, and I. M. Sebastian, “Rethink Your Approach to Digital Strategy: Experiment and Engage,” MIT Sloan CISR Research Briefing, Vol. XVIII, No. 10, October 2018.[/foot] without damaging current non-digital revenue streams or slowing their company’s digital transformation progress. But they aren’t sure what to start, stop, or continue doing.
Our past decade of data monetization research suggests that most established companies are not yet capable of monetizing data like their digital counterparts. This is because digital data monetization involves elevated analytics service quality levels, intimate customer interactions, and unintended externalities, all of which digital companies have learned how to manage. The good news: our research has identified the five data monetization capabilities that allow digital companies do this.
FIVE CAPABILITIES FOR DIGITAL DATA MONETIZATION
In 2018, MIT CISR surveyed 315 executives who were familiar with their company’s enterprise-level data activities.[foot]Wixom, “Data Monetization: Generating Financial Returns from Data and Analytics—Summary of Survey Findings.”[/foot] We studied the companies’ data monetization portfolios, capabilities, and outcomes to learn how companies are generating economic returns from data monetization activities. What emerged from this study was the clear evidence that firms with high levels of five capabilities—(1) data asset curation, (2) data factory platform, (3) data science techniques and talent, (4) customer understanding, and (5) acceptable data use—produce optimized data monetization portfolios. We know that companies that are proficient in these capabilities more successfully deploy data and analytics products, features, and experiences, and with better outcomes: the offerings are valued by customers, profitable, competitively superior, innovative, and first to market.
Data asset curation is a collection of activities that build data quality, make data consumable for end users, and prepare data for reuse. Companies with high levels of data asset curation capability appreciate that data is not a by-product of operational processes, but rather a key firm resource that must be purposely sourced, productized, and delivered. They engineer high-quality data products at exceptional service levels by applying processes and techniques that reflect “old school” manufacturing concepts like continuous improvement and control charts.
Key capability-building practices: master data management, metadata management, data integration, and data quality management
Data factory platform is purposely built software and hardware used to securely, efficiently, and pervasively ingest, transform, and disseminate data across and beyond the enterprise. Companies with high levels of the data factory platform capability can both reconfigure data to deliver data/analytics in new ways and scale data activities cost effectively.
Key capability-building practices: internal APIs, external APIs, and leading-edge data tools and techniques (e.g., cloud computing, open source database software)
Data science is the use of scientific methods, processes, algorithms, and statistics to extract meaning and insights from data. Companies with high levels of data science capability have analytics-savvy employees across the enterprise (including within the product unit) who are trained and supported to make evidence-based decisions, and who use methodologies that help them test and learn.
Key capability-building practices: reporting and dashboards, visualization, statistics, machine learning and specialized analytics, data scientist hiring and development, and data science training
Customer understanding is accurate and actionable knowledge about customer needs and behavior. Companies acquire customer understanding by collecting information through customer connections and analyzing it to extract insights about customer demographics, sentiments, context, usage, and desires. Companies with high levels of customer understanding deeply grasp customer needs and customer domain areas.
Key capability-building practices: involvement of customer-facing employees in product development, customer co-creation, and test-and-learn approaches and methodologies (e.g., A/B testing)
Acceptable data use is the use of people, data, and analytics in ways not just compliant with existing laws and regulations but also informed by organizational values and those of ecosystem actors. The risks of not using data acceptably include acting inaccurately or undesirably and not acting (when the company should act), any of which can result in financial, reputational, or constraint penalties. Acceptable data use manifests as data governance that effectively balances compliance and ethical concerns. Companies with high levels of acceptable data use capability have fluid norms and policies that effectively guide employee and partner data activities.
Key capability-building practices: employee data use oversight; partner data use oversight; customer self-management of customer data collection, use, and deletion; and data lineage management
CAPABILITIES, NOT LAKES
Companies today build data lakes, launch data science programs, and sensorize products—but many of these companies’ leaders scratch their heads at big costs and vague or unbelievable business case promises. Rest assured, these data activities will pay off—but only as well as they help build out digital data monetization capabilities. Companies that make these data investments worthwhile are ones that appreciate the capability building (and organizational learning) that the investments represent. These companies are not striving just to get better at “business intelligence;” rather, they are striving for a whole new world of market-facing data monetization activities that are yet to be identified and mastered. Consider the case of IAG.
Beginning in 2015, Insurance Australia Group Limited (IAG), the A$12 billion Australia-based insurance company, embarked on its digital journey and purposely sought to build out new data monetization capabilities.[foot]B. H. Wixom and M. L. Markus, “To Develop Acceptable Data Use, Build Company Norms,” MIT Sloan CISR Research Briefing, Vol. XVII, No. 4, April 2017.[/foot] To do this, the company established a Hadoop-based advanced analytics platform onto which it consolidated customer data from thirty operational systems; however, the data lake represented only one of many complimentary activities, including:
- Centralizing the management of customer data
- Establishing a dedicated data quality team to monitor data quality metrics and remediate them when they drop below tolerable thresholds
- Establishing a dedicated metadata team to oversee the tagging of data with contextual metadata that might indicate how and when the data was collected, its lifespan, or limitations to its use
- Acquiring forty-person analytics company Ambiata for its data science talent and analytics leadership
- Establishing a customer advocacy team to engage with and represent customers and their interests and ethical concerns
- Creating a framework regarding how IAG would collect, manage, use, and disclose customer information
- Establishing a required data extract request process for employees, to define needed data and describe its intended use, disclosure outside the company, and benefit, followed by a data governance team’s review and recommendation on whether and how to move forward
- Deepening data partner agreement reviews, contractual controls, and audits
- Over time, IAG ended up with not only a data lake but also an array of practices that contributed to the company’s strengthening digital data monetization capabilities (see figure 1).
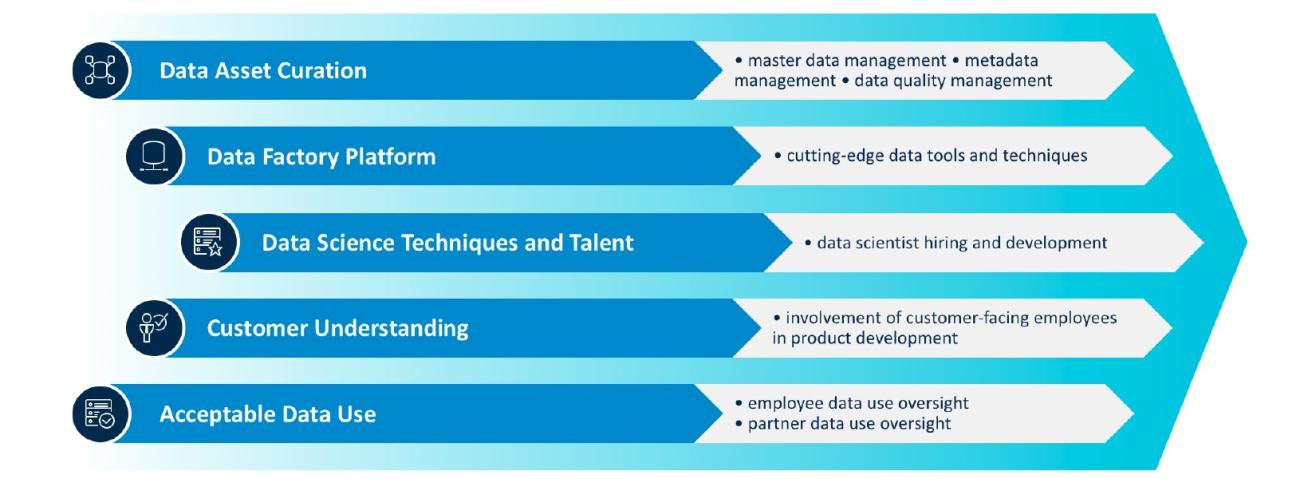
Figure 1: Building Digital Data Monetization Capabilities at IAG
Five enterprise-level capabilities combine to produce successful data monetization outcomes. A set of key practices builds each capability. The graphic illustrates the key practices in use at IAG.
THE DIGITAL DATA MONETIZATION PAYOFF
Our survey analysis showed that top-performing companies—in revenue growth, profitability, innovation, agility, and time to market—are involved in significantly more and better digital data monetization activities.[foot]The 2018 MIT CISR Data Monetization Survey (n=315). Sample was split into low-performing and high-performing companies based on self-reports of revenue growth, profitability, innovation, agility, and time to market. Comparison groups means were significantly difference using t-tests (p<.05).[/foot] And top performers are reporting that information solutions represent 20 to 26 percent of total company revenues, as compared with 12 to 14 percent for low performers.
Yes, it may feel like building data lakes, launching data science programs, and sensorizing products are taking place as behemoth, isolated initiatives. But we view these efforts as steady drumbeats on a company’s journey to become digital, and we encourage companies to use the five digital data monetization capabilities to help coordinate across their data activities. As business leaders plan and deploy data activities, they should evaluate exactly what monetization capabilities the activities build (and what data monetization returns the activities will help produce).
If you’re a business leader who wants to get a sense of your company’s potential to successfully deploy data and analytics products, features, and experiences, then we suggest you score the company on the characteristics that predicted companies with top digital data monetization performance in our survey: use of leading-edge data technologies, external APIs, use of machine learning, an analytics-savvy product unit, customer co-creation, and customer self-management of customer data.[foot]A linear regression model using survey scores from use of leading-edge data technologies, external APIs, use of machine learning, an analytics-savvy product unit, customer co-creation, customer self-management of customer data, and management views data as a strategic asset predicts the combined data monetization scores with R square of .61 (p<.000).[/foot] We know from case studies and interviews that these practices represent some of the hardest to get right—and that they enable achievement of the most elusive outcomes.
© 2019 MIT Sloan Center for Information Systems Research, Wixom and Owens. MIT CISR Research Briefings are published monthly to update the center's patrons and sponsors on current research projects.
About the Authors
MIT CENTER FOR INFORMATION SYSTEMS RESEARCH (CISR)
Founded in 1974 and grounded in MIT's tradition of combining academic knowledge and practical purpose, MIT CISR helps executives meet the challenge of leading increasingly digital and data-driven organizations. We work directly with digital leaders, executives, and boards to develop our insights. Our consortium forms a global community that comprises more than seventy-five organizations.
MIT CISR Associate Members
MIT CISR wishes to thank all of our associate members for their support and contributions.