Since 2019, MIT CISR’s data research team has followed the journeys of fifty-two AI projects.[foot]In 2019–2020, we conducted 100 interviews with 38 domain experts, 49 data scientists, and 13 consultants regarding their experiences with 52 distinct AI projects at 48 companies to learn how effectively they were achieving deployment. Of these projects, 31 were deployed, 15 were in pilot, and 6 were under development. Since then, we have followed the progress of the projects—by connecting with the project teams via calls and email—and have written case vignettes of five select initiatives.[/foot] The journeys reflect that AI initiatives grow via offshoots—like a spider plant. Through supplying the care and feeding of data and expertise, a core AI model matures into an accurate, deployable solution that the organization sustains via retraining activities and ongoing monitoring. In time, the core model spins off recontextualized models—the model repurposed in new contexts, like spider plant offshoots—that teams adapt for related solutions that serve new geographies, users, systems, decisions, subject areas, and markets. We call this pattern of model growth scaling AI, which we define as growing the value created by both a core trained model and recontextualized adaptations of the model (see figure 1).
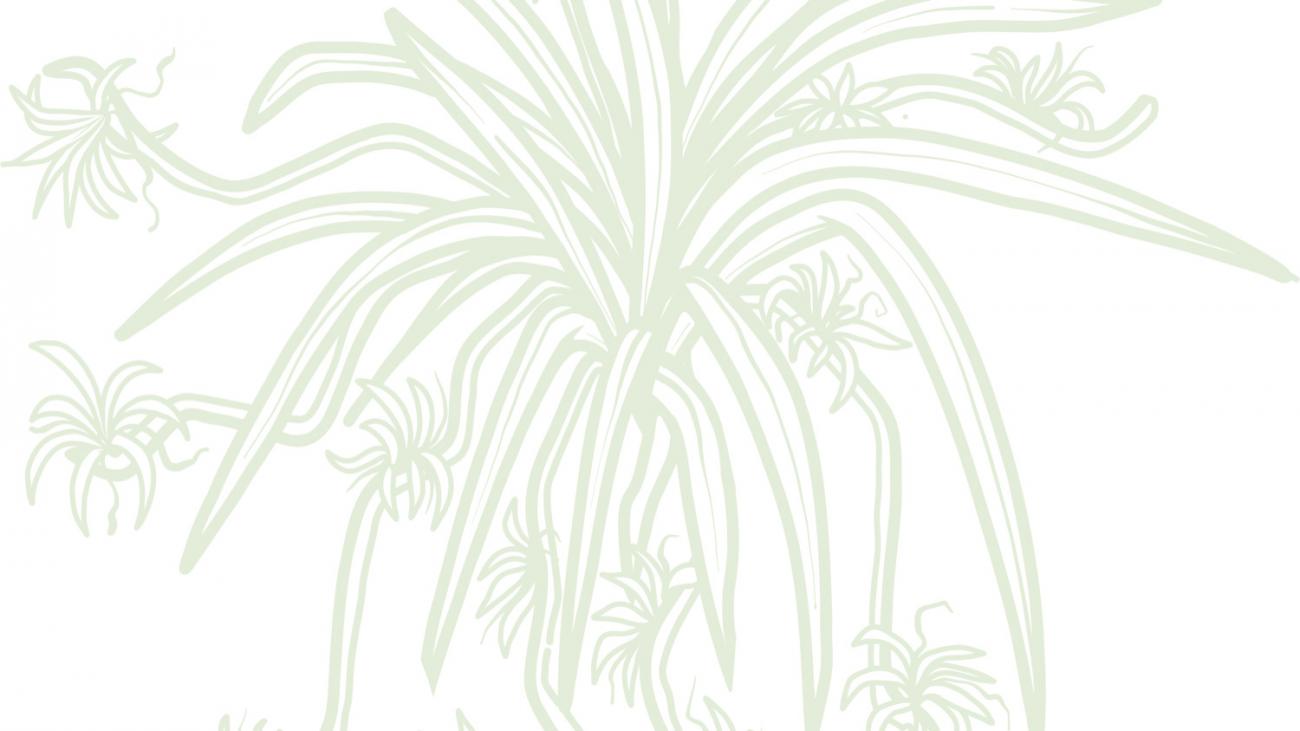
Scaling AI to Generate Better and Different Outcomes
Abstract
Since 2019, MIT CISR has investigated fifty-two AI solutions to learn about AI scaling: growing the value created by both a core trained model and recontextualized adaptations of the model. This research has identified that scaling up happens when an AI solution moves from core model development to pilot to production, with increasing value creation. Further, scaling out happens when an AI model is trained using new data and new expertise, which are required for a new related application. This briefing describes both AI scaling dimensions within the context of an AI project journey at Pegasystems.
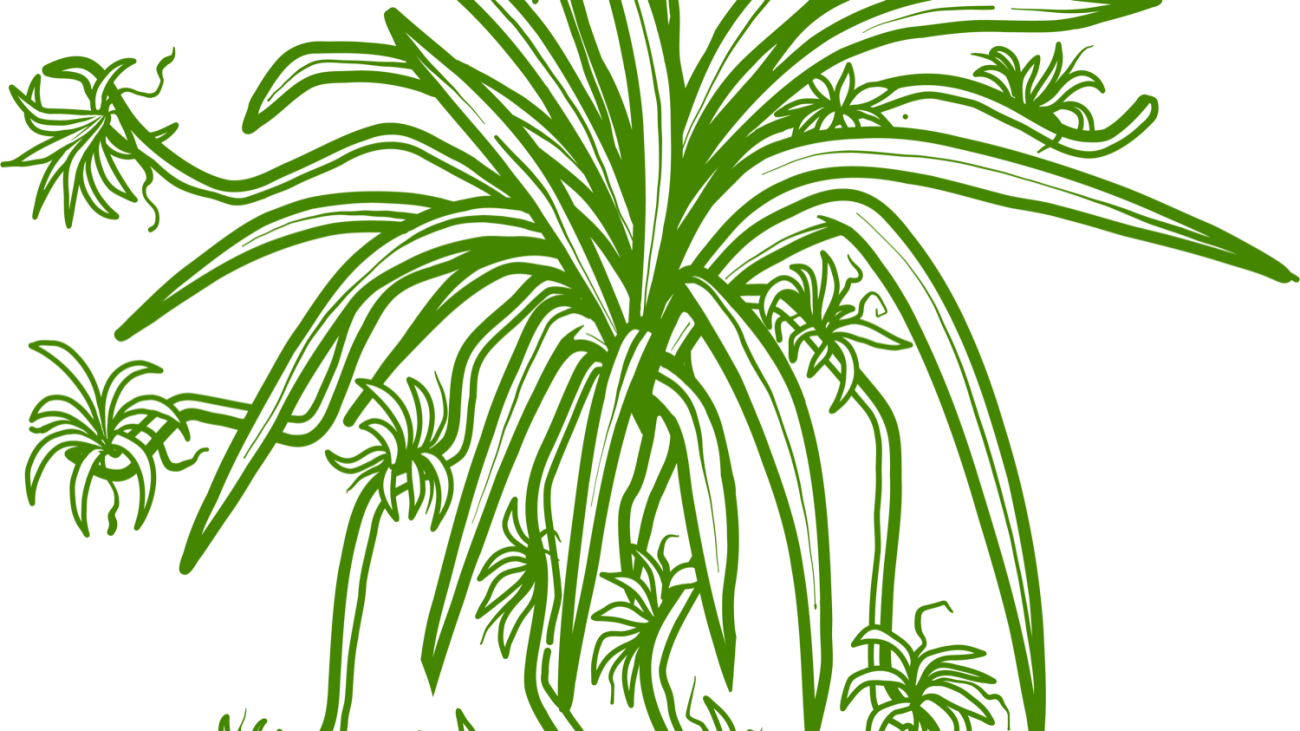
Figure 1: AI Grows Like a Spider Plant
Scaling AI means growing the value created by a core trained model and its recontextualized models.
Historically, organizations assess technology scaling using the lens of technology adoption and use; leaders have worked to maximize the number of seats, users, and tasks associated with a system investment in order to demonstrate its impact. This approach to evaluating impact falls short when it comes to scaling AI. Our research suggests that organizations manage and measure scaling AI using two distinct dimensions: scaling up and scaling out (see figure 2). We describe both dimensions in the following sections drawing from an AI project journey at Pegasystems.[foot]B.H. Wixom and C.M. Beath, “Pega: Driving Customer Engagement Using AI-Enabled Decision Making,” MIT CISR Working Paper No. 449, June 2021, https://cisr.mit.edu/publication/MIT_CISRwp449_PegaAIDecisionMaking_WixomBeath.[/foot]
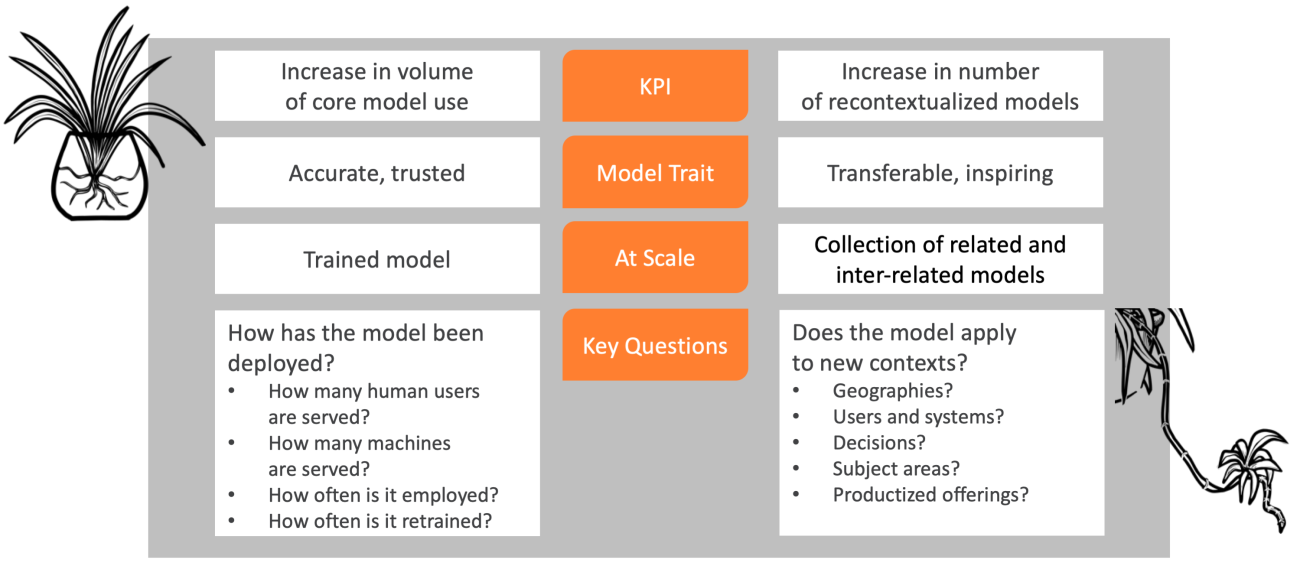
Figure 2: Scaling Up Versus Scaling Out
AI and Scaling Up
The volume of AI model use grows as the model becomes more accurate in executing its task and more trusted to perform in a desirable way. As the model’s accuracy and trustworthiness build, an organization can more confidently incorporate the model into operations. The following example illustrates that scaling AI up is a process of evolution in which both the model and the nature of work change over time. There is no end to scaling up; rather, scaling up is an ongoing management process.
Pegasystems Inc. (Pega) is a 38-year-old software company that develops and hosts software and engages in content marketing. In recent years, Pega content marketers began to see a drop in their ability to engage contacts using email blasts. In 2016, Pega leaders wondered if the company could use the proprietary AI-enabled decision-making engine it was selling for optimization of customer outreach to help Pega’s marketers optimize the company’s own customer communications. These leaders envisioned using AI to deliver individualized email outreach, identifying the next best action to take with contacts based on their varying needs and interests.
Before diving into AI model development and training, a Pega project team undertook three key foundational activities. The first activity was to build an email distribution platform that would support AI-triggered actions. The second activity was to create vetted content and tag it regarding characteristics that could inform relevance to a contact, such as the content’s language or technical complexity. And the third activity was to clean up Pega’s customer and prospect information so that it could be used for automated decision making.
Managing the Core Model
Scaling up requires that a core model be trained to support solutions that are explainable: compliant, representative, reliable, and value-generating.[foot]I. A. Someh, B. H. Wixom, C. M. Beath, and A. Zutavern, “Building an Artificial Intelligence Explanation Capability,” MIS Quarterly Executive, forthcoming.[/foot] After deployment, use of the model generates data with which the model can be retrained and improved to remain explainable. Ongoing model management ensures that the model remains aligned with an ever-shifting reality.[foot]B. H. Wixom, I. A. Someh, and R. W. Gregory, “AI Alignment: A New Management Paradigm,” MIT Sloan CISR Research Briefing, Vol. XX, No. 11, November 2020, https://cisr.mit.edu/publication/2020_1101_AI-Alignment_WixomSomehGregory.[/foot] Scaling up, in effect, represents growth in core model use.
Pega’s project team trained the AI model and identified the best criteria to predict particular customer actions. The goals of the model were to optimize content offerings, choose the best email out of hundreds in inventory to send to a specific contact, and know when to send an email. After the model was adequately trained, the team ran a pilot test of the model to match customers to email content using a small group of contacts that the company assessed to be low risk for mismarketing. The results exceeded expectations, which built confidence in the AI solution. This success encouraged the project team to run the model on the full contact database. As contacts reacted to the emails, their behaviors were captured as outcomes.
The team used the outcomes to evaluate and report on the model’s performance, and periodically to improve the next-best-action model itself. Sometimes an improvement involved adding or changing email content. At times, the AI solution proposed actions that challenged established thinking, such as matching content with an unexpected audience. In these cases, the marketers reconsidered and revised their outreach strategies.
Changing Work
Scaling up changes work because the AI solution enables new routines. Traditionally, organizations have relied on change management to drive top-down redesign of work routines and to ensure that humans will be willing and able to work in new ways. However, AI increasingly enables bottom-up changes to work routines and reengineers operations to draw on automated processes and system-to-system exchanges as well as and along with human activities. As a result, scaling up efforts often drive a decrease in human participation.
Historically, Pega marketers had strategized for email content marketing using a time-intensive process that relied on analyzing data in spreadsheets and navigating the priorities of marketers who represented different industries, functions, geographies, and offerings. This process could become uncomfortable when stakeholders had conflicting views regarding whose email should go to whom and when. The new AI solution streamlined and depoliticized content marketing by automating these decisions. This freed up marketers to spend time on more appealing work tasks. Compared to previous distribution, the AI-enabled content distribution had a different cadence, referred to as “always-on outbound.”
To recap: scaling up happens when an AI solution moves from core model development to pilot to production, with increasing value creation. The core AI model is retrained using new data and new insights related to its use case. The secret sauce for scaling up is increased model credibility and trust so that prospective users can confidently incorporate the AI solution into their work.
AI and Scaling Out
The number of an organization’s related recontextualized models grows as leaders identify new applications for a core AI model—and then apply it to new purposes. As users become more comfortable employing their AI model, they imagine novel ways it might be adapted to solve problems and fulfill unmet needs. Efforts to scale out benefit from human involvement and entrepreneurship. And more scaling up results in a greater number of people identifying other unmet needs and unsolved problems in related contexts.
The Pega story, in fact, begins with an AI "scaling out" moment when Pega marketing leaders were inspired to adapt the AI-enabled decision-making engine Pega designed for customers to optimize the company’s own processes.
Pega’s email content marketing journey also led to additional scaling out of the AI model. The project team formally presented their AI email content marketing solution across Pega to various audiences to deepen awareness and buy-in. As this use of AI became routine within the company, Pega encouraged the use of AI solutions for other similar customer-contact channels, such as the company’s website, and Pega Communities, a forum for customers using Pega products. Each new use required the email-oriented model to be recontextualized. In effect, each model recontextualization initiated a new scaling-up journey that required building accuracy and trust within the new context.
The Pega project team viewed evangelism as key to AI model repurposing and adaptation across Pega. Also, the team anticipated that model evangelism would be repeatedly necessary due to employee turnover and a constant stream of new employees who would need to understand the next-best-action concept and learn to trust Pega's AI solutions.
To recap: scaling out happens when an AI model is trained using new data and new expertise, which are required for a new related application. The model is adapted and recontextualized, with the goal of creating new opportunities to apply the model in new use cases. The secret sauce for scaling out is building model faith and enthusiasm to inspire workers to be entrepreneurial and to innovate new AI-fueled ways of working.
Scaling AI Outcomes
Yes, scaling AI is like the growth of a spider plant. Scaling up AI involves maturing AI models from pilot to deployment and beyond—like nurturing the core plant. Scaling out AI resembles when the core plant generates offshoots and thereby new growth. In a recent informal survey of the project teams of the fifty-two AI projects we followed, half of the responding teams reported both scale up and scale out progress.[foot]The research team surveyed the original 52 AI project teams in October 2021 by email regarding their progress to date with scaling their AI project. Forty-five of the 52 AI project teams replied as of publication of this research briefing. Of the represented projects, 23 had scaled up and out; 8 had scaled but only up; 2 had scaled but only out; 6 have not yet scaled; and 6 could not report progress due to team turnover.[/foot] Ideally, leaders should expect their maturing AI models to seed myriad user-inspired applications that flourish in further value-creating ways. In this way, organizations will reap not only better outcomes from maturing AI models, but also different outcomes from AI model recontextualization.
© 2021 MIT Sloan Center for Information Systems Research, Wixom, Someh, and Gregory. MIT CISR Research Briefings are published monthly to update the center's patrons and sponsors on current research projects.
About the Authors
MIT CENTER FOR INFORMATION SYSTEMS RESEARCH (CISR)
Founded in 1974 and grounded in MIT's tradition of combining academic knowledge and practical purpose, MIT CISR helps executives meet the challenge of leading increasingly digital and data-driven organizations. We work directly with digital leaders, executives, and boards to develop our insights. Our consortium forms a global community that comprises around seventy organizations.
MIT CISR Associate Members
MIT CISR wishes to thank all of our associate members for their support and contributions.